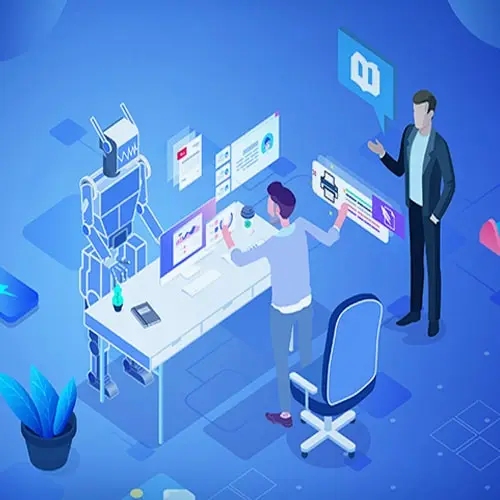
Synthetic data is rapidly becoming a game-changer in the field of AI, offering a myriad of benefits for training AI models.
Using synthetic or artificially generated data in training AI algorithms is a burgeoning practice with significant potential. It can address data scarcity, privacy, and bias issues and raise concerns about data quality, security, and ethical implications.
See how synthetic data works wonders:
# Data Abundance: Real-world data can be costly, scarce, or even impossible to acquire due to privacy concerns. Synthetic data provides a virtually unlimited and customizable source of data, enabling you to train your models on diverse scenarios and edge cases.
# Bias Mitigation: Real-world data can often be biased, leading to biased AI models. Synthetic data allows you to control the distribution of data and inject specific characteristics, mitigating biases and promoting fairer models.
# Improved Generalizability: Synthetic data lets you create datasets with specific variations and anomalies, helping your models generalize better to real-world scenarios they haven't encountered before.
# Faster Development Cycle: With ample synthetic data, you can iterate on your models faster and experiment with different training methodologies without being limited by real-world data restrictions.
# Privacy Protection: Sensitive data can be anonymized or replaced with synthetic equivalents, protecting privacy while still enabling valuable AI development.
# Cost Reduction: Collecting and cleaning real-world data can be expensive. Synthetic data offers a cost-effective alternative, especially for tasks requiring large datasets.
There are some of the ways synthetic data can benefit AI development. As the technology matures and becomes more sophisticated, we can expect even more exciting applications in various fields, from healthcare and finance to autonomous vehicles and robotics.
Secondly, when synthetic data can do wonders in training AI models, it can certainly become research’s poster boy. As it stands it can only happen in conjunction with real data. A *data mapping* is a must today to take a call whether a doctoral or post-doctoral research proposition would lead to a few original findings or not.
The validation of real data is no mean task and it has to start from there. Otherwise, it is likely to vitiate the subsequent process of the creation of synthetic data. Synthetic data creation / usage as an input is a complex interdisciplinary task and can happen only at the level of a specific sector or even lower, depending on the complexity of that sector. Only then it becomes an objective and empirical research input.
See What’s Next in Tech With the Fast Forward Newsletter
Tweets From @varindiamag
Nothing to see here - yet
When they Tweet, their Tweets will show up here.