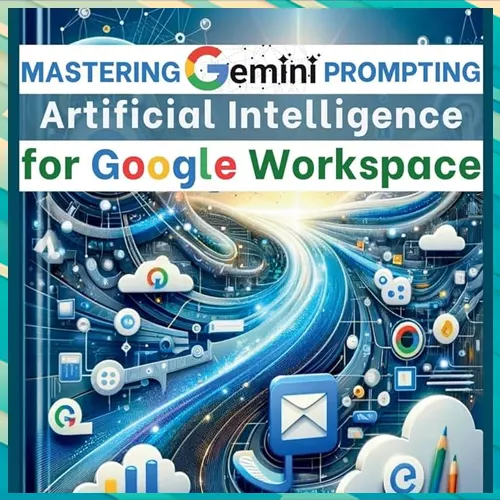
As businesses move beyond trial-and-error with AI tools like ChatGPT and Claude, Google’s guide promotes consistency through clear examples, concise prompts, and direct instructions—key strategies to enhance reliability using few-shot prompting techniques
With generative AI tools becoming more powerful and widely adopted, the art of writing effective prompts—known as prompt engineering—has emerged as a specialized and essential skill. In response to the growing need for structured approaches in this field, Google has released a comprehensive 68-page white paper aimed at helping developers and enterprise users get the most out of its Gemini model, available through Vertex AI and a public API. Authored by software engineer Lee Boonstra, the guide brings together academic research and real-world testing from Google’s own AI labs to offer a systematic approach to prompt design.
Mastering prompt accuracy
Prompt engineering is about crafting precise input text that guides large language models (LLMs) toward accurate, coherent, and contextually appropriate responses. While early users of tools like ChatGPT and Claude often relied on trial and error, businesses today seek consistency and dependability. Google's guide addresses this by highlighting the importance of using clear, direct examples to establish patterns (a method known as few-shot prompting), keeping prompts concise, and giving explicit instructions rather than relying on vague or negative directions. These methods are designed to reduce ambiguity and enhance the quality of the AI's output.
Strategic prompting for AI efficiency
The white paper also emphasizes the strategic use of different types of prompts. System prompts are used to define the AI’s overarching role, such as instructing it to act as a financial advisor, while contextual prompts provide background information relevant to the specific task, such as describing a client's risk profile. The guide advocates for techniques like “chain of thought” prompting, where the AI is encouraged to reason step-by-step by thinking aloud—an approach shown in studies to improve multi-step problem-solving. Other key strategies include managing token limits to control the length and clarity of responses, reusing variables to reduce repetition and prompt length, and requesting structured outputs like JSON to streamline integration with other software applications.
Standardizing AI prompting techniques
Although the guide is centered around Google’s Gemini models, its insights are applicable across other major LLM platforms, including ChatGPT, Claude, and Meta’s LLaMA. Experts have welcomed the release as a meaningful step toward standardizing prompt engineering. Dr. Marissa Lee, an AI consultant, noted that clearer prompting not only improves the quality of responses but also reduces the risk of AI hallucinations—where the model generates convincing but incorrect information. As AI becomes increasingly embedded in areas like customer service, content creation, and data analysis, tools like Google’s prompt guide are becoming essential for ensuring accuracy, reliability, and responsible use.
See What’s Next in Tech With the Fast Forward Newsletter
Tweets From @varindiamag
Nothing to see here - yet
When they Tweet, their Tweets will show up here.